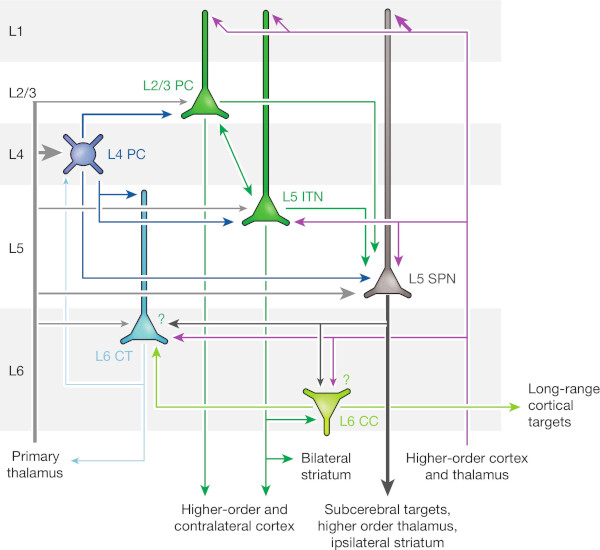
In [1] we approach the question whether the columnar structure of the cortex reflects a common computational principle by focusing on a putative core function of the cortical minicolumn that is implemented by a specific subset of neurons while leaving the overall function of the entire minicolumn unspecified for now. Given the canonical connectivity of cortical principal cells shown in figure 1, we argue that groups of layer 2/3 and upper layer 5 neurons ( L2/3 PC and L5 ITN in Fig. 1) form a local, autoassociative memory that builds the core functionality of a minicolumn while all other neurons depicted in figure 1 serve supporting functions, e.g., communication to subcerebral targets ( L5 SPN ), communication to long-range cortical targets ( L6 CC ), modulation of sensory input or input from lower-order cortex ( L6 CT ), or modulation of the autoassociative memory itself ( L4 PC ). We base this hypothesis on earlier work directed at modeling and understanding of grid cells located in layer 2/3 and layer 5 of the medial entorhinal cortex.
So far the grid cell model describes a single group of neurons that is able to learn a sparse, distributed representation of its input space. As such, the group acts as a local, input-driven transformation that maps input signals to output signals without maintaining an active state, i.e., if no input is present, no output will be generated. However, if two groups share the same main input space but also receive the group activity of each other as an additional input signal, both groups are able to maintain a stable active state even when the main input signal vanishes. Instead of being stateless input-output transformations the two groups form an active autoassociative memory cell (AMC) [3,4]. Given the canonical connectivity of cortical principal cells shown in figure 1 we argue that L2/3 PC and L5 ITN constitute such a pair of reciprocally connected neuron groups forming an AMC. Both groups share a main input space via connections from the primary thalamus and local L4 PC while they also both receive feedback input at their distal dendrites in layer 1 from higher-order cortex and the thalamus.
The other neuron groups shown in figure 1 can be interpreted as providing support for the AMC: The outputs of both L2/3 PC and L5 ITN connect locally to L5 SPN, which projects to subcerebral targets, e.g., motor centres, and non-locally to higher-order parts of the cortex. The output of the lower group L5 ITN makes an additional local connection to L6 CC, which projects to long-range cortical targets, but also locally to L6 CT, which in turn connects back to L4 PC and the primary thalamus. We propose that the described neural structure constitutes a single cortical minicolumn. At its core the minicolumn hosts a single autoassociative memory cell ( L2/3 PC / L5 ITN ) that is supported by neurons regulating its activity ( L4 PC ), its input ( L4 PC / L6 CT ) and its output ( L5 SPN / L6 CC ).
A key property of the described AMC is its ability to feed its output back into itself via the reciprocal connections between the two neuron groups ( L2/3 PC / L5 ITN ). This local feedback loop does not only allow the AMC to stay active when the main input vanishes. It also enables a form of attractor dynamics [3,4] where an initial, potentially noisy or ambiguous input pattern can be iteratively refined to approach one of the stable, prototypical input patterns learned by the AMC. In addition, this iterative process can be supported or modulated by the feedback input integrated via the distal dendrites of both neuron groups. On a cortex-wide level these attractor dynamics might play an important role to dynamically bind subsets of cortical minicolumns into joint, temporarily stable attractor states.
The outlined cortical column model suggests that the cortex may consist of a network of autoassociative memory cells that influence each other via feedback as well as feedforward connections while trying to achieve locally stable attractor states. In addition, further cortical circuitry may modulate the activity of individual cortical columns to facilitate competition among cortical columns or groups of cortical columns on a more global level, which may then lead to the emergence of stable, global attractor states that are able to temporarily bind together sets of cortical columns.
References
1
,
A Grid Cell Inspired Model of Cortical Column Function,
In: 10th International Joint Conference on Computational Intelligence (IJCCI 2018), Seville, Spain, September 18-20., pp. 204–210, 2018,
[pdf|doi|bibtex]
2
,
Cortical connectivity and sensory coding,
In: Nature, 503:51, 2013,
[doi]
3
,
Sparse Distributed Memory,
In: MIT Press, Cambridge, MA, USA, 1988,
[details]
4
,
Sparse distributed memory and related models,
In: Research Institute for Advanced Computer Science, NASA Ames Research Center, 1992,
[details]