All multicellular life requires some form of coordination between the cells that make up an organism. This fundamental requirement was met by evolution with an abundance of biochemical signaling mechanisms that gave rise to ever more intricate and complex patterns of interaction. For example, the release of signaling molecules during development is a key factor in the self-organized assembly of the growing organism. Biochemical signals guide the migration of cells and control their differentiation. Throughout life, local diffusion of signaling molecules communicates events like injury or infection to nearby cells while the release of signaling molecules into circulatory systems allows to broadcast information more widely. All these signaling mechanisms have in common that specific molecules encode specific messages or information in their given context. They have a fixed meaning that is encoded in DNA and can, for the most part, be only updated through the slow process of evolution. With the advent of nervous systems this changed dramatically.
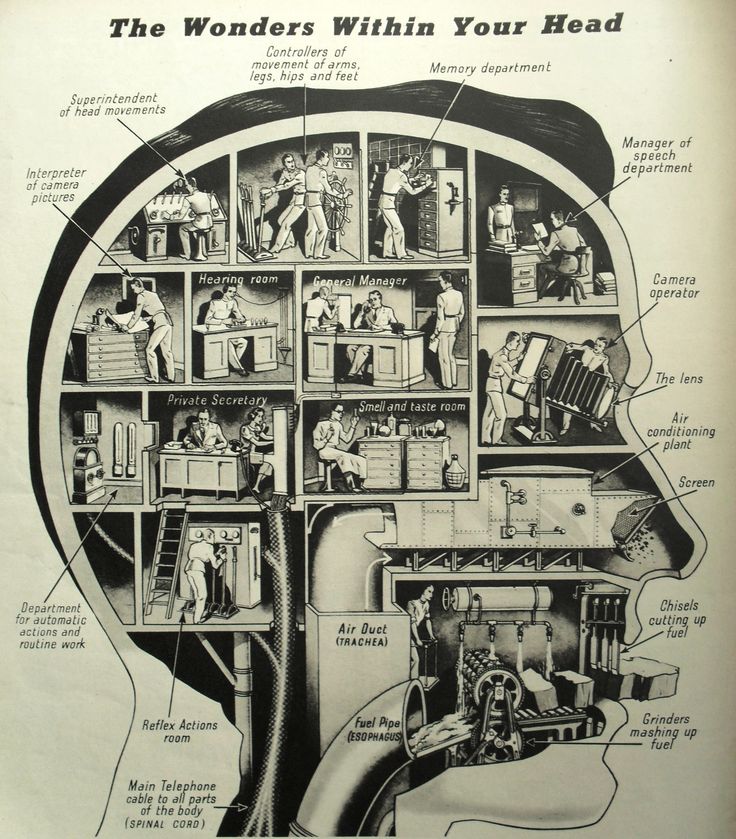
A nervous system utilizes the existing forms of signaling mechanisms in a completely new way. Local diffusion is reduced to tiny gaps between the cells that increase the precision and speed of communication between them. To complement this extreme localization, the cells themselves developed the ability to grow projections that can reach up to several meters in some organisms transporting signals on the inside via fast signaling cascades. The guidance of these projections, globally as well as locally, utilizes mechanisms akin to those that guide the self-assembly during the development of the organism. Together, the increase in speed and localization of signals and the ability to form a network of cells that is not only shaped by information encoded in DNA but also by the signals themselves enables organisms to recognize patterns, i.e., to recognize the repeated simultaneity of signals by connecting the corresponding cell projections with a common receiving cell. This way, patterns present in a set of signals get represented in physical form within a network of cells and the behaviour of the cells within this network is now dominated by these patterns rather than the individual signals. Thus, the advent of nervous systems opened up a new layer of information processing, a new layer of abstraction, that is built on and integrated with the underlying biochemical signaling systems. It is the first abstraction in information processing of many. Transforming a multicellular organism into an animal that displays behavior. An animal that eventually starts communicating and coordinating with others, expanding the level of information processing beyond the indvidual into ever more complex flows of information and respective interaction at a now social level. Further levels of abstraction may follow including the invention of artifacts to encode information in the physical world and the development of artifacts that can process the former entities on their own.
If you look around you’ll see a world that is to a large degree the result of these increasingly abstract flows of information and their processing. Trying to understand how nervous systems work is to some extent the attempt to understand the first step in this cascade of abstractions. Due to their size, complexity, and tight integration the investigation of nervous systems is an incredibly challenging task. Within the last 150 years the scientific community gathered a vast amount of data on this topic and gained significant understanding of how the different parts of a nervous system work and how they interact on a basic level. However, despite these advances there is still no coherent theory that would explain how the observable, macroscopic behavior of an organism emerges from the interaction of these different parts. One main reason for this is the difficulty of observing a sufficiently large part of the nervous system in detail while it is operating. Currently, we can either observe the activity of a small amount of neurons with great detail or we see the average activity of large groups of neurons from a global perspective. In both cases, a common experimental approach is the correlation of external stimuli with the measured activity of the neurons or neuronal regions under investigation. A classic example of this approach is the work of Hubel and Wiesel who measured the activity of neurons in the first layers of the cat’s visual cortex in response to simple, visual stimuli [1,2]. The main drawback of this method is that it usually can only be applied to the early layers of a cortical region. Later stages of cortical processing integrate so much additional information that the neuronal activity of these deep layers cannot be correlated with external stimuli any more. Yet, in 2004 May-Britt and Edvard Moser discovered a type of neuron that represents a rare exception to this rule [3,4]. They found neurons in the entorhinal cortex of rats whose activity correlates strongly with the absolute position of the animal in its environment. Moreover, the activity of these so-called grid cells correlates not only with one but multiple locations that form a highly regular, hexagonal pattern spanning the entire environment. The entorhinal cortex is the gateway between neocortex and hippocampus and it receives highly processed information. Thus, entorhinal grid cells offer the rare opportunity to study the activity of neurons at a late stage of cortical processing while still being able to correlate their activity with an external variable (the animal’s location). May-Britt and Edvard Moser received a Nobel Prize for their discovery in 2014.
My own research on grid cells focused on finding a computational model that could describe the peculiar behavior of these cells [5,6,7,8,9,10,11,12,13,14]. I discovered that the behavior of grid cells can be described as a special case of a general computational principle. With this novel model I was able to replicate a wide range of grid cell related phenomena and, moreover, could begin to formulate the hypothesis that the underlying computational principle might apply to neuron groups throughout the entire cortex [15,16]. My research regarding the latter hypothesis is still ongoing.
References
1
,
Receptive fields of single neurones in the cat's striate cortex,
In: The Journal of Physiology, 148, 574-591, 1959,
[doi]
2
,
Receptive fields, binocular interaction and functional architecture in the cat's visual cortex,
In: The Journal of Physiology, 160, 106-154.2, 1962,
[doi]
3
,
Spatial Representation in the Entorhinal Cortex,
In: Science, volume 305, pages 1258-1264, number 5688, 2004,
[doi]
4
,
Microstructure of a spatial map in the entorhinal cortex,
In: Nature, volume 436, pages 801-806, number 7052, 2005,
[doi]
5
,
A Computational Model of Grid Cells Based on Dendritic Self-Organized Learning,
In: Proceedings of the International Conference on Neural Computation Theory and Applications, 2013,
[pdf|bibtex]
nominated for best paper award
6
,
A New View on Grid Cells Beyond the Cognitive Map Hypothesis,
In: 8th Conference on Artificial General Intelligence (AGI), 2015,
[pdf|doi|bibtex]
7
,
Noise Resilience of an RGNG-based Grid Cell Model,
In: Proceedings of the 8th International Joint Conference on Computational Intelligence, IJCCI 2016, Volume 3: NCTA, Porto, Portugal, November 9-11, pp. 33–41, 2016,
[pdf|doi|bibtex]
nominated for best paper award
8
,
Modelling the Grid-like Encoding of Visual Space in Primates,
In: Proceedings of the 8th International Joint Conference on Computational Intelligence, IJCCI 2016, Volume 3: NCTA, Porto, Portugal, November 9-11, pp. 42–49, 2016,
[pdf|doi|bibtex]
best paper award
9
,
A Computational Model of Grid Cells based on a Recursive Growing Neural Gas,
In: PhD thesis. University of Hagen, 2016,
[pdf|bibtex]
awarded with the Fakultätspreis 2017 for the best scientific work 2016 at the faculty of mathematics and computer science at the University of Hagen
10
,
Entorhinal Grid Cells May Facilitate Pattern Separation in the Hippocampus,
In: Proceedings of the 9th International Joint Conference on Computational Intelligence, IJCCI 2017, Funchal, Madeira, Portugal, November 1-3, pp. 141–148, 2017,
[pdf|doi|bibtex]
11
,
Simulation von Gitterzellen als Spezialfall eines allgemeinen neuronalen Verarbeitungsprinzips,
In: Springer–Verlag, 2018,
[bibtex]
"Informatik Spektrum" cover
12
,
A Survey of Entorhinal Grid Cell Properties,
In: arXiv e-prints, arXiv:1810.07429 (Oct. 2018), arXiv:1810.07429. arXiv: 1810.07429 [q-bio.NC], 2018,
[pdf|bibtex]
13
,
A Possible Encoding of 3D Visual Space in Primates,
In: Computational Intelligence: International Joint Conference, IJCCI 2016 Porto, Portugal, November 9–11, 2016 Revised Selected Papers. Cham: Springer International Publishing, pp. 277–295, 2019,
[pdf|doi|bibtex]
14
,
A Noise Compensation Mechanism for an RGNG-Based Grid Cell Model,
In: Computational Intelligence: International Joint Conference, IJCCI 2016 Porto, Portugal, November 9–11, 2016 Revised Selected Papers. Cham: Springer International Publishing, pp. 263–276, 2019,
[pdf|doi|bibtex]
15
,
A Grid Cell Inspired Model of Cortical Column Function,
In: 10th International Joint Conference on Computational Intelligence (IJCCI 2018), Seville, Spain, September 18-20., pp. 204–210, 2018,
[pdf|doi|bibtex]
16
,
Challenging the Intuition About Memory and Computation in Theories of Cognition,
In: Proceedings of the 11th International Joint Conference on Computational Intelligence - Volume 1: NCTA, (IJCCI 2019). INSTICC. SciTePress, pp. 522–527, 2019,
[pdf|doi|bibtex]